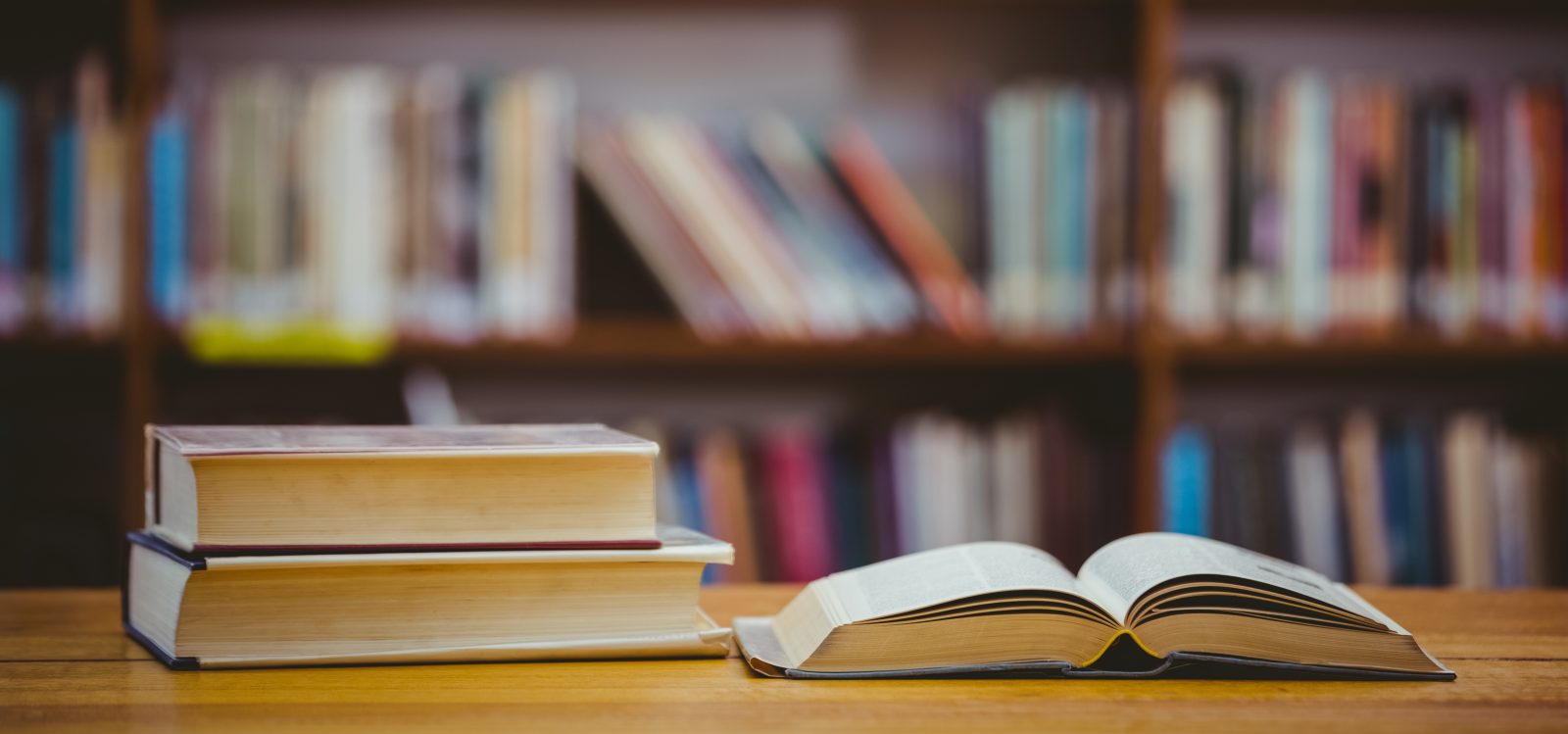
Menstrual Cycle
CHAPTER ONE INTRODUCTION 1. 0 BACKGROUND OF THE STUDY Menstrual cycle is a process experienced by every female at one point in their life cycle. In the olden days a young lady experiences her first menstruation in her late teens or early twenties. In recent times, however, some begin their menstrual cycle as early as nine years. The age at menarche (the first time a girl or a young woman menstruates) is widely considered as an important landmark in sexual maturity. However it varies widely between the same people.
These variations has been attributed to a number of possible causes such as; advancement in technology, health care delivery, weather and climate change, food, daily activities, socio-economic activities, lifestyle and many more. The importance of the role of menstrual cycle in every lady’s life is paramount. The menstrual cycle enables you to mark out certain activities like, when to time pregnancy, safe periods, expected menstruation days, and also proves when you get pregnant.
Keeping track of one’s menstrual cycle enables one to discover health problems which may arise in time due to a change in menstrual cycle pattern. Knowledge about ones menstrual cycle is not naturally known from birth. A research conducted by students from the University of Jammu indicated that, out of a sample of 200 females from a rural area, only a few respondents showed accurate and adequate knowledge about menstrual cycle. Menstrual cycle is the monthly process of ovulation and menstruation that occurs between puberty and menopause in women and female primates who are not pregnant (Encarta Dictionary).
The key processes are ovulation and menstruation. In the first half of the menstrual cycle, levels of estrogen rise and make the lining of the uterus grow and thicken. In response to follicle-stimulating hormone, an egg (ovum) in one of the ovaries starts to mature. At about day 14 of a typical 28-day cycle, in response to a surge of luteinizing hormone, the egg leaves the ovary. This is called ovulation. In the second half of the menstrual cycle, the egg begins to travel through the fallopian tube to the uterus.
Progesterone levels rise and help prepare the uterine lining for pregnancy. If the egg becomes fertilized by a sperm cell and attaches itself to the uterine wall, the woman becomes pregnant. If the egg is not fertilized, it either dissolves or is absorbed into the body. If pregnancy does not occur, estrogen and progesterone levels drop, and the thickened lining of the uterus is shed during the menstrual period. Menstruation is the monthly process of discharging blood and other matter from the womb that occurs if fertilization does not take place.
The menstrual blood is partly blood and partly tissue from the inside of the uterus (womb). It flows from the uterus through the small opening in the cervix, and passes out of the body through the vagina. Most menstrual periods last from three to five days. It is of utmost importance in the reproductive physiology of females. The term is derived from the Latin word ‘menses’ meaning month, and Greek word ‘mene’ meaning moon, with reference to the fact that ‘lunar month’ is approximately 28 days long, and a complete menstrual cycle usually takes about 28 days.
Menstrual cycles are often irregular through adolescence, particularly the interval from the first to the second cycle. According to the World Health Organization’s international and multicenter study of 3073 girls, the median length of the first cycle after menarche was 34 days, with 38% of cycle lengths exceeding 40 days. Variability was wide: 10% of females had more than 60 days between their first and second menses, and 7% had a first cycle length of 20 days. Most females bleed for 2 to 7 days during their first menses. Early menarche is associated with early onset of ovulatory cycles.
When the age at menarche is younger than 12 years, 50% of cycles are ovulatory in the first gynaecologic year (year after menarche). By contrast, it may take 8 to 12 years after menarche until females with later-onset menarche are fully ovulatory. Despite variability, most normal cycles range from 21 to 45 days, even in the first gynaecologic year, although short cycles of fewer than 20 days and long cycles of more than 45 days may occur. Because long cycles most often occur in the first 3 years post menarche, the overall trend is toward shorter and more regular cycles with increasing age.
By the third year after menarche, 60% to 80% of menstrual cycles are 21 to 34 days long, as is typical of adults. An individual’s normal cycle length is established around the sixth gynaecologic year, at a chronologic age of approximately 19 or 20 years. Two large studies, one cataloguing 275947 cycles in 2702 females and another reporting on 31645 cycles in 656 females, support the observation that menstrual cycles in girls and adolescents typically range from 21 to approximately 45 days, even in the first gynaecologic year. In the first ynaecologic year, the fifth percentile for cycle length is 23 days and the 95th percentile is 90 days. By the fourth gynaecologic year, fewer females are having cycles that exceed 45 days, but anovulation (the state of not ovulating because of a medical condition, suppression by drugs or menopause) is still significant for some, with the 95th percentile for cycle length at 50 days. By the seventh gynaecologic year, cycles are shorter and less variable, with the fifth percentile for cycle length at 27 days and the 95th percentile at only 38 days.
Thus, during the early years after menarche, cycles may be somewhat long because of anovulation, but 90% of cycles will be within the range of 21 to 45 days. Irrespective of utmost importance of menstruation in women, and the growth end existence of generations, there are many misconception, superstitions, taboos and myths associated with menstruation that have not been flattering to womankind. The misconceptions pave way for certain unhygienic practices which allows easy access to contracting reproductive tract infections.
The major cause of these misconceptions is the lack of education, awareness, personal and social inhibitions in discussing such matters. In keeping with the important role of menstruation in reproductive health of women, the present study was conducted to evaluate the relationship between the age, interval of menstrual cycle and the duration of menstruation. The study seeks to find whether one can accurately depend on age, interval of cycle or duration of menses to make assumptions or predictions about ones menstrual cycle and other factors associated with it. . 1. STATEMENT OF THE PROBLEM. There are always misconceptions about the menstrual cycle of females. Some of these misconceptions are mythical or traditional. Other misconceptions are due to sheer lack of knowledge about menstruation and its association with the female populace. The misconceptions often lead to unexpected problems; diseases, unplanned pregnancies, unhygienic practices, ostracism, dejection and many more. Menstrual cycles and it’s related topics are seen as extremely delicate and hence not an issue to talk about in everyday conversation.
From our interview with respondents, we gathered information about how people use their age and other conditions or factors to make predictions; safe and unsafe periods, when to expect next menstruation period etc. The research therefore seeks to evaluate the reliability of this information. The aim of this project is to statistically analyze information on menstrual cycles to assess the relationship that exists between the age of a respondent, the duration of her menses and length or interval for each cycle.
Statistical tools will be employed to analyze the data and bring out meaningful information. 1. 2. OBJECTIVES OF THE STUDY The main objective of this study is to determine whether there is a relationship between age, duration of menses and the cycle of each female. The specific objectives include: a. To determine whether the average interval within cycles and menses are the same across the two age groups. b. To determine whether interval of cycle depend on age c. To determine whether we can classify the age of a person based on the duration and interval within cycle d.
To determine whether duration of menses depend on age 1. 3. RESEARCH QUESTIONS i. What does the personal body rhythm which determines the length of menstrual cycle depend on? ii. Is age a factor that affect the menstrual cycle? iii. Is the duration of menstruation dependent on the length of one’s cycle? iv. Are age, duration of menstruation and length of menstrual cycle related? 1. 4. LITERATURE REVIEW This section of the study takes a look at research studies on menstrual cycle carried out by other people.
In a study carried out by America Academy of Pediatrics (2006) in the United States of America on Girls and Adolescence on the topic: ‘Menstruation in Girls and Adolescents; Using Menstrual Cycle as a Vital Sign. Their main objective was to be able to educate young patients and their parents regarding what to expect of a first period and about the range for normal cycle length of subsequent menses. Also for clinicians to have an understanding of bleeding patterns in girls and adolescents, the ability to differentiate between normal and abnormal menstruation, and the skill to know how to evaluate young patients’ conditions appropriately.
Assessing both the normal and abnormal menstruation, they observed that menstrual cycles are often irregular through adolescence, particularly the interval from the first to the second cycle. According to the World Health Organization’s international and multicenter study of 3073 girls, the median length of the first cycle after menarche was 34 days, with 38% of cycle lengths exceeding 40 days. Variability was wide: 10% of females had more than 60 days between their first and second menses, and 7% had a first cycle length of 20 days. Most females bleed for 2 to 7 days during their first menses.
Despite the variability, most normal cycles range from 21 to 45 days, even in the first gynecologic year, although short cycles of fewer than 20 days and long cycles of more than 45 days may occur. They concluded that since menarche is such an important milestone in physical development, it is important to be able to educate young females and their parents regarding what to expect of a first period and about the range for normal cycle length of subsequent menses. A study was also conducted by Yan Liu et’all (1991) to evaluate factors affecting menstrual cycle characteristics (American Journal of epidemiology, volume 160, issue 2, Pp. 31 – 140). This study in California and Utah used daily urinary metabolites of estrogen and progesterone and computer algorithms to assess ovulatory status and day of ovulation. They examined the associations of risk factors with menstrual cycle characteristics for 309 working women aged 20–44 years who collected a median of five cycles each of daily urine samples. Linear mixed models were used to assess continuous menstrual outcomes. Compared with women less than age 35 years, women aged 35 years or older had a significantly decreased (–0. 94 days, 95% confidence interval: –1. 3, –0. 05) adjusted mean cycle length. Age modified the effects of smoking, physical activity, ethnicity, and alcohol consumption on mean follicular phase length. Asian women had a significantly longer (1. 65 days, 95% confidence interval: 0. 54, 2. 76) adjusted mean cycle length compared with Caucasian women. Compared with women who did not consume alcoholic drinks, women who consume alcoholic drinks had a significantly shorter (–1. 26 days, 95% confidence interval: –2. 21, –0. 31) adjusted mean cycle length. Mean cycle and phase lengths were ignificantly associated with length of the prior luteal phase. These results indicate that potentially modifiable risk factors, as well as immutable host factors, are associated with menstrual cycle characteristics that may in turn be related to subsequent disease risk. There are forums being held all over the world concerning the menstrual cycle patterns. One of such forums was held at the New York Academy of Sciences by leading women’s health advocates and co-sponsored by Rachel’s Well, Inc. and the Society for Menstrual Cycle Research. Rachel’s Well, Inc. s a non-profit organization that aims to improve access to health services for uninsured women and develop a model of healthcare delivery that will improve women’s health. The organization was founded by Dr. Lawrence Nelson, research gynaecologist, and a dedicated group of professionals, who feel that there is a need for increased capacity to provide preventative healthcare services for women. Rachel’s Well, Inc. initiated the development of “The Menstrual Cycle is a Vital Sign” to establish an educational platform through which the role of menstruation as a unique indicator of a woman’s overall physical health can be communicated.
The organization hopes to create a model of healthcare delivery that could have a significant impact on policy-making decisions and improve the health of women. (http://www. rachelswell. org) and the Society for Menstrual Cycle Research is also a non-profit organization founded by a multidisciplinary group of women who were pioneers in understanding the centrality of menstrual cycle research to women’s health. The organization consist of researchers, health care providers, policy makers, and students who share an interest in women’s lives and health needs as they are related to the menstrual cycle.
Their mission is to be the source of guidance, expertise, and ethical considerations for researchers, practitioners, policy makers and funding resources interested in the menstrual cycle. They do this by offering a network of communication and support that spans discipline, professional responsibilities, and geography to provide woman-centred perspectives on menstrual experiences. (http://www. pop. psu. edu/smcr). The discussion was designed to raise awareness about the role of menstruation as a unique indicator of a woman’s overall physical health.
At the centre of the discussion was the importance of identifying health issues linked to the menstrual cycle, as well as clarifying any misconceptions. At the forum, Paula Hillard, M. D. , professor of obstetrics ; gynaecology and paediatrics at the University Of Cincinnati College Of Medicine said, “The menstrual cycle is a window into the general health and well-being of women, and not just a reproductive event” and it can indicate the status of bone health, heart disease, and ovarian failure, as well as long-term fertility. Therefore, if a woman is not having her period, it is the first sign that something else could be going on. Doctors and scientists also discussed the long- and short-term effects of irregular or interrupted periods (amenorrhea) brought on by over-training of female athletes and excessive dieting and exercise, as well as oral contraceptives designed to suppress the monthly cycle. The experts called for more research to determine the impact of menstrual suppression and its potential side effects on future fertility and long-range health. “The most important thing to emphasize about menstrual suppression is that the long-term effects are simply unknown,” said Jerilynn Prior, M.
D. , professor of endocrinology and metabolism at the University of British Columbia in Vancouver, and board member for the Society for Menstrual Cycle Research. “Whether a woman is electing to do so with new birth control products, or it results from excessive dieting or exercise, we as a society are allowing the one vital sign unique to women to go unmonitored, which could ultimately lead to an enormous uncontrolled experiment with a woman’s health. ” The clear message of the forum was the importance of the relationship between women and their menstrual cycles. Regular and effective health screenings and an open dialogue between women and their health care providers are critical to their overall health,” said Lawrence Nelson, M. D. , M. B. A. president of Rachel’s Well, Inc. 1. 5. DATA COLLECTION The data were collected from primary source. The primary data were obtained from selected female students of the University of Cape Coast. The sampling method used is the simple random sampling method and we employed questionnaires which were distributed randomly to females all over campus after interview with them. No priorities were given to students of any level.
Interviews were used as a way of familiarizing the respondent with the questionnaire which is to be answered. The interview also sought to inquire about the general perception of respondents about menstruation and its related issues and to encourage respondents to give accurate information for the purpose of the research. The questionnaires were then administered to the respondents. Both methods were used so that more information could be obtained. The data were sequences of records made about the menstrual cycles of the females regularly for a period of three months and also the duration of each menstruation.
The data also included the ages of respondents. Due to the nature of our target population, most of the ages of respondents fell between the ages of 19 and 26 and only a few extreme values of which the highest age was 29 were obtained. The dates for the beginning of each menstruation for the three month period were obtained from the questionnaires and manual calculations were made to acquire the different cycle lengths of each respondent since that was the major information needed from the dates provided. The results were then tabulated. A copy of this is seen at the Appendix B. . 6. OUTLINE OF THE STUDY In this study, five main chapters are considered. Chapter One looks at the introduction which includes background of the study, statement of the problem, objectives of the study, research questions, literature review, data collection and outline of the study. Chapter Two reviews basic methods that are used to analyze the data; these include multivariate analysis of variance, regression analysis and discriminant analysis. Chapter Three talks about preliminary analysis of the data, and this include tables, graphs and cross tables.
Chapter Four present further analysis of the data. This includes multivariate analysis of variance (MANOVA), regression analysis and discriminant analysis. Finally, chapter Five talks about the summary, findings and conclusions. CHAPTER TWO REVIEW OF BASIC METHODS 2. 0. INTRODUCTION In this chapter, the methods that will be used in the analysis of the data to achieve the objectives of this study are reviewed. The statistical methods that are used are; the multivariate analysis of variance test and the discriminant analysis. 2. 1. MULTIVARIATE ANALYSIS OF VARIANCE
Analysis of variance is used when we are confronted with two variables that are related to each other. It can also be used when experimental or predictor variables are qualitative in nature. Comparisons of the means of two populations can be extended to the comparison of three or more populations. In the case of three or more means, an appropriate procedure that can be used is called analysis of variance. When data involves several multivariate means, a much suitable technique is the multivariate analysis of variance (MANOVA). MANOVA is a generalized form of the univariate analysis of variance.
It is used when there are two or more dependent variables. Multivariate analysis of the variance makes use of positive-definite matrices whose diagonal entries are the same kinds of sums of squares that appear in univariate analysis of variance. MANOVA is based on the product of model variance matrix and the error variance matrix inverse. MANOVA statistics should be a measure of magnitude of the singular value decomposition of this matrix product, but there is no unique choice owing to the multi-dimensional nature of the alternative hypothesis. The most common statistics are * Samuel Stanley-Wilks’ lambda ? The pillai-M. S Bartlet trace * The Lawley -Hoteling trace. * Ray’s greatest root. In the case of two groups, all the statistics are equivalent and the test reduces to Hotelling’s T-square test. The structure of a multivariate data can be presented as shown below VARIABLESvar 1var 2var p1x11x21xp1ITEMS2x12x22xp2? n? x1n? x2n? xpn Where xi is the measurement of the ith variable on the jth item. i starts from 1 to p and j starts from 1 to n. Due to the nature of this study, we shall limit ourselves to the multivariate analysis of variance. 2. 2.
MULTIVARIATE ANALYSES OF VARIANCE (MANOVA) Data used for multivariate analysis of variance should be of three or more populations treating the same variables. The sample size in each population can vary. The samples for various populations are assumed to be independent and all populations have a common covariance matrix ?. Each population is multivariate normally distributed. The general model of the multivariate analysis of variance is given as Model:Xij=? +? j+? ij,i = 1,2,…,n and j = 1,2,…,k, where Xijis the ithunit from the jthtreatment with the respective means µj and the common variance ? . µ is referred to as the grand mean. ?jis called the treatment effects and they are such that ? j=0. The mean of the jthtreatment is written as ? j=? +? jand imposed the condition ? j=0 so that the mean of the ? jequals the grand mean?. ?ij are the error terms, which are the values of nk=N independent random variables having normal distributions with zero means and the common variance ? 2. The model can be partitioned as follows Xij= X+Xj-X+Xij- Xj where X is the overall mean, Xj – Xis the effect due to treatment between groups and Xij-Xj is the random error within groups.
It can be shown through algebraic manipulations that i=1niXij-X2=j=1gnjXj -X2+i=1gj=1njXij-Xj2………* Where X=i=1njj=1kXijN Is the grand mean. In equation * B = Sum of square between (treatment) = i=1gnjXi-XXi -X? With degree of freedom g – 1, where g is the number of populations W = Sum of squares within = i=1gj=1njXij-XiXij-Xi? With degree of freedom j=1gnj-g B + W = Total sum of squares = i=1gj=1njXij-XXij-X? With degree of freedom j=ignj-1 2. 3. THE TEST STATISTIC Multivariate analysis of variance tests the hypothesis Ho:? 1=? 2=…=? j against the alternative
H1:? i?? j where i? j Some of the test statistics used includes the Wilks lambda approach and the Bartlett’s approach. The Wilks lambda is given by ? *=WB+W It is also known as the ratio of generalized variances. For this kind of approach, Ho is rejected when the ratio is too small. The Bartletts approach makes use of the following relation k=-n-1-p+g2ln? * k=-n-1-p+g2lnWB+W This equation is approximately chi – square distributed with p (g – 1) degree of freedom, where g is the number of populations and p is the number of variables and n is the number of observations.
For the Bartletts approach, we reject H0 at significance level ? , if k is greater than ? 2pg-1 The multivariate analysis of variance can be summarized in a table called multivariate analysis of variance (MANOVA) table as shown in the table below. Source of variation| Matrice of sum of squares and cross products| Degree of freedom| Mean sum of squares| F – statistic| Treatment (between)Residual (within)| SStr (B)SSw (W)| g -1i=1gni-g| SStrg-1SSwi=1gni-g| MStrMSw| Total| SST (W+B)| i=1gni-1| | | 2. 4. ASSUMPTIONS OF THE MODEL * Sample size The normality assumption * The linearity assumption * . Homogeneity of variance and covariance * . Outliers * Multicollinearity and Singularity 2. 5. DISCRIMINANT ANALYSIS Discriminant Function Analysis (DA) undertakes the same task as multiple linear regressions by predicting an outcome. When three or more categories are involved, the technique is referred to as multiple discriminant analysis. DA involves the determination of a linear equation like regression that will predict a categorical variable. The multiple case requires more than one function. 2. 6.
THE MODEL The discriminant analysis model involves linear combinations of the following form: D=b0+b1X1+b2X2+b3X3+? +bkXk Where D = discriminant score bs = discriminant coefficients or weights Xs = predictors or independent variables The weights (b) are estimated so that the groups differ as much as possible on the values of the discriminant function. Discriminant function analysis is broken into a 2-step process: * Testing significance of a set of discriminant functions, and; * Classification.
The first step is computationally identical to MANOVA. The multivariate test is first performed, and, if statistically significant, proceeds to see which of the variables have significantly different means across the groups. Classification of variables is undertaken when group means are found to be statistically significant. Discriminant analysis automatically determines some optimal combination of variables so that the first function provides the most overall discrimination between groups, the second provides second most, and the functions do not overlap.
Subjects are classified in the groups in which they had the highest classification scores. 2. 7. COMPUTATIONS Fundamental equations for DA are the same as for MANOVA: Cross-products matrices for between-groupbg differences and within – groupswg differences are first created as SSTotal=SSbg+SSwg The determinants are calculated for these matrices and used to calculate a test statistic (using either Wilks’ Lambda or Pillai’s Trace). Wilks’ Lambda follows the equation: ? =SSwgSSwg+SSbg Next an F ratio is calculated as in MANOVA: Fappdf1,df2=1-yydf2df1
For cases where n is equal in all groups: y=? 1s , s=p2k-12-4p2+k-12-5 , p = number of predictor variables dferror=n-1k and dfeffect=k-1 , where k is the number of groups The degrees of freedom (1 and 2) are given by df1=pdfeffect df2=sdferror-p-dfeffect+12-pdfeffect-22 For unequal n between groups, this is modified only by changing the dferrorto equal the number of data points in all groups minus the number of groups (N –k). If the experimental F exceeds a critical F, then the experimental groups can be distinguished based on the predictor variables.
The number of discriminant functions used in the analysis is equal to the number of predictor variables or the degrees of freedom, whichever is smaller. Classification functions are derived from the linear discriminant functions. For cases with an equal sample size for each group the classification function coefficient Cjis equal to the sum of: Cj=Cj0+Cj1X1+Cj2X2+? +CjpXp for the jthgroup, j=1? k,X= raw scores of each predictor,Cj0 = a constant. If W = within – group variance-covariance matrix, and M = column matrix of means for group j, then the constant Cjo=-12CjMj. For unequal sample size in each group: Cj=Cj0+i=1pCijXj+lnnjN j= size in group j, N = total sample size. 2. 8. Assumptions Discriminant function analysis is computationally very similar to MANOVA, and all assumptions for MANOVA apply as explained above. * Sample size * Normal distribution * Homogeneity of variances/covariances * Outliers * Multicollinearity This analysis makes use of the Fisher’s discriminant functions for separation and classification. Fisher’s discriminant analysis allows for the following: * Convenient representations of the g populations that reduce the dimension from a very large number of characteristics to a relatively few linear combinations. Plotting of the means of the first two or three linear combinations * Scatter plots of sample values of the first two discriminants, which can indicate outliers or other abnormalities The primary purpose of Fisher’s discriminant analysis is to separate populations, however it can also be used to classify. CHAPTER THREE PRELIMNARY ANALYSIS 3. 0. INTRODUCTION This chapter presents the descriptive analysis of the data and the preliminary assumption test for MANOVA and discriminant analysis which was conducted to check for normality, linearity, outliers, homogeneity of variance-covariance matrices and multicollinearity.
Two dependent variables were used; interval between cycles and duration of menses. The independent variable was Age interval. 3. 1. DESCRIPTIVE ANALYSIS This considers the basic statistics of the variables. Table 3. 1 describes the central tendencies and dispersion. TABLE 3. 1: Descriptive Statistics | ————————————————- Item meanmedianvarianceminimummaximum ————————————————- Duration mean 4. 60574. 66670. 7053. 337. 00 ————————————————- Cycle interval mean 27. 243928. 33339. 18820. 031. 67 The basic statistics shows that the mean duration of menses for each of the 82 females have a mean of 4. 6, which is approximately 5 days. This implies that on the average a female menstruates for about 5 days. Their mean cycle interval is also approximately 27 days which also implies that the average interval within a typical cycle is 27 days. The results also shows that variability is very low within the duration of menses and very high within the cycle interval. From our data the minimum and maximum observations for the duration of menses are 3 and 7 days respectively. The minimum and aximum observed values for the interval within cycle were also 20 and 32 days respectively. 3. 2. TEST FOR MANOVA AND DISCRIMINANT ANALYSIS ASSUMPTIONS INTRODUCTION: This section deals with the testing of the various assumptions that are needed to be checked to ensure the feasibility of MANOVA and Discriminant Analysis. 3. 3. TABLE 3. 2: Normality assumption | Kolmogorov-Smirnov| | Statistic| Df| Sig. | Durmean| . 117| 82| . 007| cycintmean| . 196| 82| . 000| It was observed after performing a test of Normality for duration of menses and interval within cycle that, there was a significant test after using the Kolmogorov-Smirnov test.
This indicates that the normality assumption was violated for both duration of menses and interval within cycle. This is because a p-value of 0. 007 and 0. 000 respectively, which is lower than the ? level of 0. 05. FIGURE 3. 1: Linearity Assumption To test for this assumption, a matrix of scatterplot was generated between each pair of variables separately for each age interval. These plots did not show any obvious sign of non-linearity. Therefore, the linearity assumption has been satisfied. 3. 4. Figure 3. 2: Outliers To check for univariate outliers, a box and whisker plot was generated and there were no extreme values.
This indicates that, the univariate outlier assumption was satisfied. We also check for multivariate outliers using the Mahalanobis Distance. We compare Mahalanobis Distance’s maximum value with chi-square value with the number of dependent variables and the degree of freedom with ? -value of 0. 001. The maximum value of Mahalanobis distance from our analysis is 8. 157 which is less than the chi-square value of 13. 82. This means that there were no multivariate outliers. Therefore, the multivariate outlier’s assumption was satisfied. 3. 5. Multicollinearity Assumption TABLE 3. : Correlations| | Duration of menses| Interval between cycle| Spearman’s rho| | Duration of menses| 1. 000| . 059| | | Interval between cycle| . 059| 1. 000| To check for the multicollinearity assumption, a pair wise correlation between the dependent variables was generated. Spearman’s rank correlation was used since the dependent variables violated the normality assumption. There was a very weak correlation between the dependent variable about 0. 059. This shows that the multicollinearity assumption was violated. 3. 6. Homogeneity of variance-covariance matrices TABLE 3. : Box’s Test of Equality of Covariance Matrices. Box’s M| . 431| F| . 140| df1| 3| df2| 263779. 505| Sig. | . 936| Since the significant value, 0. 936, is greater than 0. 001, we have not violated the equality of variance-covariance matrices. CHAPTER FOUR 4. 0. INTRODUCTION In view of the data and other information provided on the data, an attempt is made to use the statistical too, discussed in Chapter Two to further analyze the data to achieve the specific objective set in this study. Several tests will be conducted to verify the observations made about the data in relation to our objective.
Based on the results of the preliminary analysis, we observed that the normality assumption and the multicollinearity assumption were violated. The other assumptions on the other hand were satisfied. 4. 1. Multivariate Analysis of Variance ( MANOVA) A one-way between-groups multivariate analysis of variance was performed to investigate whether there was difference in the interval between cycles and duration of menses across the Age groups. Two dependent variables were used; interval between cycles and duration of menses. The independent variable was Age interval (below 25years and 25years and over).
Preliminary assumption testing was conducted to check for the various assumptions. The test indicates that the normality assumption and the multicollinearity assumption were violated. The other assumptions on the other hand were satisfied thus the test on MANOVA was successful. Since we have tested for the assumptions of MANOVA, we now compare the population mean for duration of menses and intervals between cycles do not vary across females below 25years and females 25years and over. HYPOTHESIS Ho: the population mean for duration of menses and interval between cycles do not vary across females below 25years and females 25years and over.
H1 :The population mean for duration of menses and interval between cycles varies across females below 25years and females 25years and over From SPSS analysis, we obtained a pillai’s trace value of 0. 091, with a significance value of 0. 023. This is less than 0. 05; therefore there is significant difference between the population mean for duration of menses and interval between cycles across females below 25years and females above 25 years. This indicates that females in different age categories have different cycle interval and different menstrual duration.
Thus females that happen to be in the same age category tend to have similar cycle interval and menstrual duration. TABLE 4. 2 This table shows how females below 25 and females above 25 differ on the dependent variable (age interval). ————————————————- SourceDependent VariabledfFSig. ————————————————- ————————————————- Age intervalDuration of menses10. 1290. 721 ————————————————- Interval between cycle 18. 0440. 006 ————————————————- ErrorDuration of menses80 ———————————————— Interval between cycle80 From our conclusion of the Pillai trace significant value, we want to reduce the chance of type I error and hence we apply the Bonferroni adjustment as a follow up test to ascertain the validity of the conclusion. We will therefore compare the significant values of the Bonferroni adjustment with ? -level of 0. 025. There was a significant difference for the interval between cycles with significance 0. 006 which is less than 0. 025. Therefore the conclusion was valid and we found the difference to be coming from the interval between cycles.
Therefore we can say that females in different age categories differ in their interval between cycles (the number of days between the three cycles). 4. 2. Discriminant Analysis Discriminant function analysis is multivariate analysis of variance (MANOVA) reversed. In MANOVA, the independent variables are the groups and the dependent variables are the predictors. In discriminant analysis, the independent variables are the predictors and the dependent variables are the groups. As previously mentioned, discriminant analysis is usually used to predict membership in naturally occurring groups.
It answers the question: can a combination of variables be used to predict group membership? Usually, several variables are included in a study to see which ones contribute to the discrimination between groups. Discriminant function analysis is in two phases: (1) testing significance of a set of discriminant functions, and; (2) classification. The first step is computationally identical to MANOVA. The two matrices are compared via multivariate F tests in order to determine whether or not there are any significant differences (with regard to all variables) between groups.
One first performs the multivariate test, and, if statistically significant, proceeds to see which of the variables have significantly different means across the groups. TABLE 4. 4 : Classification Function Coefficients| | age in interval| | below 25| 25 and over| Durmean| 5. 369| 5. 387| cycintmean| 2. 975| 3. 194| (Constant)| -52. 390| -58. 484| | The Fisher’s linear discriminant functions obtained from the above table are given as follows: F1=5. 369D+2. 975C-52. 390 and F2=5. 387D+3. 194C-58. 484 These functions show how the population was classified.
The first function provides the overall most discrimination between the age groups and the second provides the second most discrimination between the age groups. TABLE 4. 3 : Wilks’ Lambda| Test of Function(s)| Wilks’ Lambda| Chi-square| df| Sig. | 1| . 909| 7. 573| 2| . 023| To test the significance of the set of discriminant function, we compare the significance value of the wilks’ lambda to alpha (? ) value of (0. 05). From our test, the significance value of 0. 023 is less than the alpha value of 0. 05 i. e. (0. 023<0. 05), thus discriminant analysis can be used.
TABLE 4. 5 : Classification Results| | | age in interval| Predicted Group Membership| Total| | | | below 25| 25 and over| | Original| Count| below 25| 24| 25| 49| | | 25 and over| 7| 26| 33| | %| below 25| 49. 0| 51. 0| 100. 0| | | 25 and over| 21. 2| 78. 8| 100. 0| The classification results shows that out of 49 females below the age 25, 49% (24 ladies) of them were correctly classified and 51% (25 ladies) of them were wrongly classified. Also out of 33 females above 25, 21. 2 %( 7 ladies) were wrongly classified and 78. 8% (26 ladies) were correctly classified.
This implies that 26 females above the age of 25 were classified with slightly better accuracy (78%) than ladies below the age of 25, with slightly better accuracy (49%). In general 61% of the sample was correctly classified, which shows the accuracy of discriminant analysis. CHAPTER FIVE SUMMARY, DISCUSSIONS AND CONCLUSION 5. 0 INTRODUCTION In this chapter our main emphasis is on the outcome of the analysis. It also considers the extent to which the objectives of the study have been achieved. This chapter includes the summary, discussions and conclusion. 5. 1 SUMMARY
Menstrual cycles and it’s related topics are seen as extremely delicate and hence not an issue to talk about in everyday conversation. From our interview with respondents, we gathered information about how people use their age and other conditions or factors to make predictions; safe and unsafe periods, when to expect next menstruation period etc. The research therefore seeks to evaluate the reliability of this information. The study was conducted to determine whether one’s menstrual cycle depends on her age. This was done because of some challenging issues some ladies within the same age group face.
Various research has been conducted over the years to help answer some of the problems encountered by females. In a study carried out by America Academy of Pediatrics (2006) in the United States of America on Girls and Adolescence on the topic: ‘Menstruation in Girls and Adolescents; Using Menstrual Cycle as a Vital Sign. Their main objective was to be able to educate young patients and their parents regarding what to expect of a first period and about the range for normal cycle length of subsequent menses. It is believed that females within the same age group tend to face certain similar issues during menstruation.
The study explored the three cycles with a period of three months, determined the interval within cycle, the duration of menses and the age of the respondents. The data which was collected was basically primary in nature, based on the on-set of cycle, end of cycle, the duration of menses, and age of female students of the University Cape Coast. A one-way between-groups multivariate analysis of variance was performed to investigate whether there was difference in the interval between cycles and duration of menses across the age groups.
Two dependent variables were used; interval between cycles and duration of menses. The independent variable was Age interval (below 25years and 25years and over). From the study, some assumptions were satisfied and others were violated. The analysis indicated a statistical significant difference between females under 25years and females 25years and over. An inspection of the mean scores indicated that females 25years and over had slightly higher interval between cycles (M = 28. 354, SD = 0. 506) than females under 25 (M = 26. 496, SD = 0. 415).
Discriminant analysis was performed as a follow up test to ascertain how the groups differ on the composite of the dependent variables and to show how they are related. Two independent variables were used; interval between cycles and duration of menses. The dependent variable was Age interval (below 25years and 25years and over). Preliminary assumption test conducted for discriminant analysis showed same results as that of the MANOVA. The follow up test was used to classify the ladies into two age groups. The classification showed that 61% of the ladies were correctly classified, which shows the accuracy of discriminant analysis.
Thus one can use the combination of the cycle interval to predict the age group of a female but we cannot depend on the duration of the menses entirely to also determine the age of a female. 5. 2 DISCUSSIONS Menstrual cycle and its related topics are seen as extremely delicate issues which are not often discussed in our society. From our interview with respondents, we observed that people use their age other conditions like interval within cycle to make predictions such as; safe and unsafe periods for pregnancy.
The analysis of the study shows that the ladies in different age categories tend to have different duration of menses and interval between cycles. Thus using age and interval within cycle to make predictions about safe and unsafe periods for pregnancy would be reliable. Interestingly the study also reveals that, one can be classified into a particular age category based on their duration of menses and interval between cycles. Based on our findings, we recommend that: Females can use their ages to make predictions about the length of their cycles but not the durations of their menses.
They can also use their cycles to make predictions about their sexual and reproductive health. However, females are advised not to rely fully on their cycles since there can be variations in the regularity of their cycles. 5. 3CONCLUSIONS From the analysis of the study, the following conclusions were drawn: Generally, the study showed that the average interval between cycle vary across the two age groups. Females in the same age categories happen to have similar cycle interval, thus interval between cycles depends on age. Females in the same age categories can be classified based on the duration and interval between cycles.
Finally the duration of menses does not depend on age because ladies in different age categories have similar duration of menses. REFERENCE 1. Kachigan S. K. (1982). Multivariate Statistical Analysis. 1st edition. New York : Radius Press. 2. Liu Y. et all (1991). Factors Affecting Menstrual Cycle Characteristics, American Journal of Epidemiology, vol. 160, issue 2, Pp. 131 – 140. 3. Harlow S. D, Matanosla G. M. (1991). The Association Between Weight, Physical Activity and Stress and Variation in the Menstrual Cycle, American Journal of Epidemiology, vol. 133. Pp 38- 49. 4.
Gordor, B. K and Howard, N. K. (2006). Introduction to Statistical Methods. Ghana Mathematics Group. 5. Trivedi U. N et’al. (2011). Women and their menstrual cycle awareness survey, IJPI’s Journal of Hospital and Clinical Pharmacy. Retrieved February 20, 2011, from http://www. ijpijournals. com. 6. Gosline A. (2007). Do women who live together menstruate together menstruate together? Retrieved January 28,2011. 7. National women’s health information center (2006). Menstruation and the Menstrual Cycle. Retrieved February 20,2011, from http://www. 4women. org. APPENDIX A Questionnaire
Please tick [v] where appropriate 1. YEAR OF STUDY 100[] 200[] 300[] 400[] 2. FACULTY………………………………………………………… 3. AGE……………………………………………….. 4. OCCUPATIONAL STATUS : EMPLOYED[]SELF EMPLOYED[] STUDENT[]UNEMPLOYED[] 5. PLACE OF RESIDENCE HALL[] AMAMOMA[]APEWOSIKA[] KWAPROW[]OTHERS[](SPECIFY)…………………………… Please fill in the following as applicable 6. HAVE YOU HAD YOUR PERIOD OVER THE LAST FOUR MONTHS?
YES [] NO [] 7. DO YOU REMEMBER THE DATES OF YOUR MENSES? YES [] NO [] If you answered YES to the above question please continue from question 8 to 11. If you answered NO to the above question please continue from question 11 to 16 8. PLEASE FILL IN THE DATES FOR THE ONSET OF EACH CYCLE DATES JANUARY ………………………………………………….. FEBRUARY ………………………………………………….. MARCH …………………………………………………..
APRIL ……………………………………………………. 9. PLEASE FILL IN THE DATES FOR THE END OF EACH CYCLE DATES JANUARY ………………………………………………….. FEBRUARY ………………………………………………….. MARCH ………………………………………………….. APRIL ……………………………………………………. 10. WHAT IS THE DURATION OF EACH MENSTRUAL PERIOD 3 DAYS[]4 DAYS[]5 DAYS[]OTHER[] (PLEASE SPECIFY)…………………………………………. 1. WHAT CYCLE ARE YOU ON 14 DAYS[]21 DAYS[]28 DAYS[]NO IDEA[] 12. DO YOU REMEMBER THE DATE OF YOUR LAST PERIOD? YES[]NO[] 13. WHEN DID IT START? ……………………………………………………………. 14. WHEN DID IT END? ………………………………………………………………. 15. WHAT CYCLE ARE YOU ON 14 DAYS[]21 DAYS[]30 DAYS[] NO IDEA [] TABLES Code Book Age 1 = Below 25 2 = 25 years and above