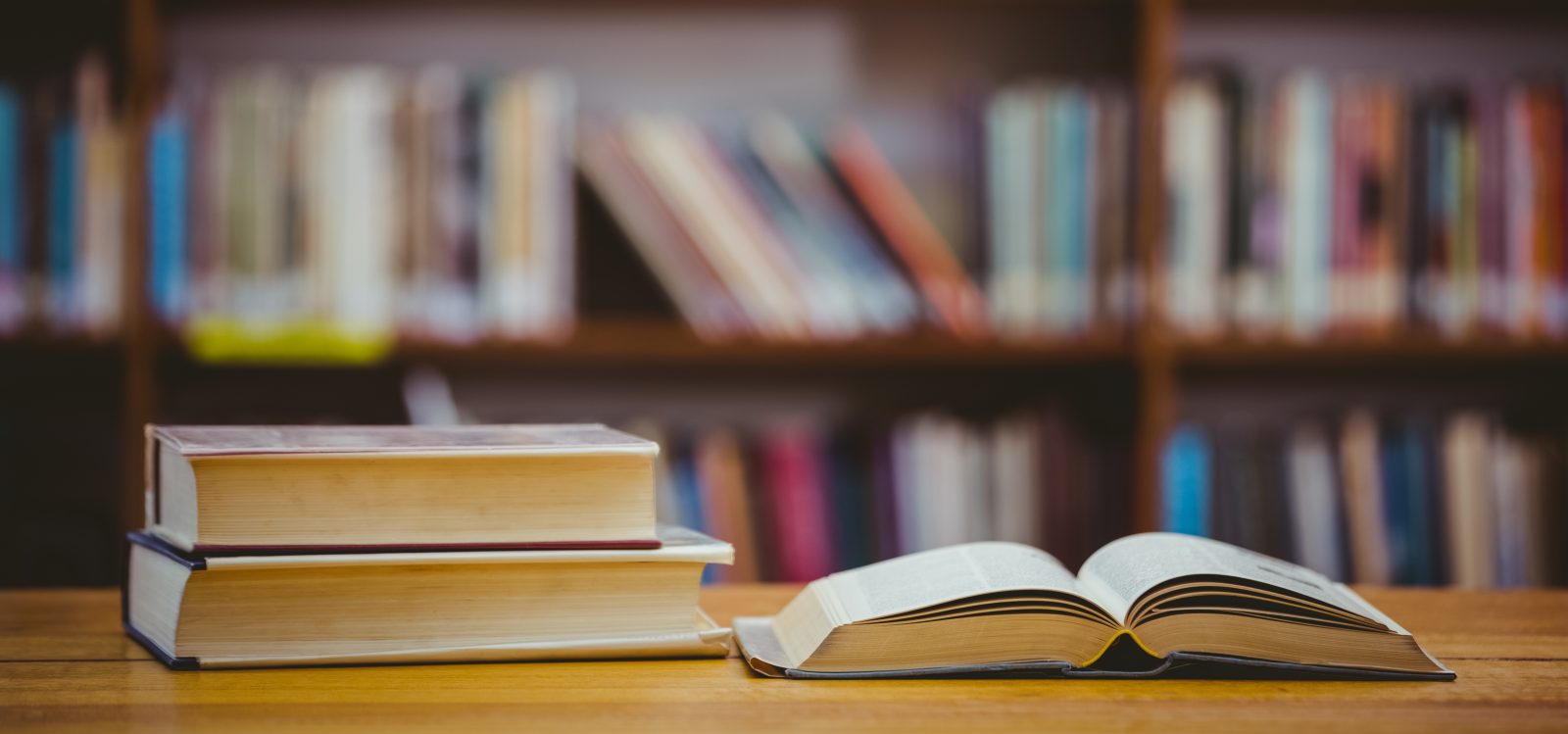
Value of Imperfect Information
Value of Imperfect Information 20 20. 1 PRIOR PROBLEM BEFORE INFORMATION Technometrics, Inc. , a large producer of electronic components, is having some problems with the manufacturing process for a particular component. Under its current production process, 25 percent of the units are defective. The profit contribution of this component is $40 per unit. Under the contract the company has with its customers, Technometrics refunds $60 for each component that the customer finds to be defective; the customers then repair the component to make it usable in their applications.
Before shipping the components to customers, Technometrics could spend an additional $30 per component to rework any components thought to be defective (regardless of whether the part is really defective). The reworked components can be sold at the regular price and will definitely not be defective in the customers’ applications. Unfortunately, Technometrics cannot tell ahead of time which components will fail to work in their customers’ applications. The following payoff table shows Technometrics’ net cash flow per component. Figure 20. Payoff Table Component Condition Good Defective Technometrics’ Choice Ship as is Rework first +$40 +$10 -$20 +$10 What should Technometrics do? How much should Technometrics be willing to pay for a test that could evaluate the condition of the component before making the decision to ship as is or rework first? 238 Chapter 20 Value of Imperfect Information Figure 20. 2 Solution of Prior Problem and EVPI EVPI $7. 50 Ship as is $25. 00 No add’l info 1 $25. 00 EVUU Rework first $10. 00 $10. 00 1. 00 Good $40. 00 Ship as is 2 $32. 50 0. 75 “Good” 1 $40. 00 Rework first $10. 00 $10. 00 Perfect info $32. 50 EVPP Ship as is -$20. 0 0. 25 “Defective” 2 $10. 00 Rework first $10. 00 $10. 00 1. 00 Defective -$20. 00 -$20. 00 0. 00 Good $40. 00 $40. 00 $40. 00 0. 00 Defective -$20. 00 -$20. 00 $40. 00 -$20. 00 0. 25 Defective -$20. 00 0. 75 Good $40. 00 $40. 00 20. 2 Imperfect Information 239 Figure 20. 3 EVPI Sensitivity to Prior Probability EVPI Sensitivity to Prior Probability, P(Good) $16 $14 $12 $10 EVPI $8 $6 $4 $2 $0 0. 0 0. 1 0. 2 0. 3 0. 4 0. 5 0. 6 0. 7 0. 8 0. 9 1. 0 Prior Probability, P(Good) 20. 2 IMPERFECT INFORMATION An engineer at Technometrics has developed a simple test device to evaluate the component before shipping.
For each component, the test device registers positive, inconclusive, or negative. The test is not perfect, but it is consistent for a particular component; that is, the test yields the same result for a given component regardless of how many times it is tested. To calibrate the test device, it was run on a batch of known good components and on a batch of known defective components. The results in the table below, based on relative frequencies, show the probability of a test device result, conditional on the true condition of the component. Figure 20. Likelihoods Test Result Positive Inconclusive Negative Component Condition Good Defective 0. 70 0. 10 0. 20 0. 30 0. 10 0. 60 For example, of the known defective components tested, sixty percent had a negative test result. An analyst at Technometrics suggested using Bayesian revision of probabilities to combine the assessments about the reliability of the test device (shown above) with the original assessment of the components’ condition (25 percent defectives). 240 Chapter 20 Value of Imperfect Information This method of assigning probabilities helps to keep the two uncertainties separate.
The main event of interest is the condition of a component, either Good or Defective. The information event of interest is the result of the test, either Positive, Inconclusive, or Negative. Technometrics uses expected monetary value for making decisions under uncertainty. What is the maximum (per component) the company should be willing to pay for using the test device? When Technometrics has the option to test the component before deciding whether to ship as is or rework, this test portion of their decision problem has the following structure. Figure 20. Structure of the Test Alternative good ship as is defective positive rework first good ship as is defective test inconclusive rework first good ship as is defective negative rework first The probabilities we assign to the event branches must be consistent with the prior probabilities of the condition of the component and the likelihoods regarding test results (conditional on the state of the component). Gigerenzer (2002) suggests that most people find it easier to determine the appropriate probabilities by expressing the data as frequencies as shown below. 20. Imperfect Information 241 Figure 20. 6 Expressing Prior and Likelihoods as Frequencies 525 positive 750 good 150 inconclusive 75 1,000 chips 25 positive negative 250 defective 75 inconclusive 150 negative Consider 1,000 chips. To be consistent with P(Good) = 0. 75, 750 are good, and 250 are defective. Of the 750 good chips, to be consistent with the likelihood P(Positive|Good) = 0. 70, 70% of the 750 good chips, i. e. , 525, have a test result of Positive. The other frequencies on the right side of the figure are consistent with the priors and likelihoods.
But the order of the events for this display of input data is the opposite of the order of events for the actual decision problem. For the actual decision problem, we need P(Positive). That is, if we decide to test, we first see the uncertain test result. From the frequencies, we note that 525 good chips test positive and 25 defective chips test positive. Since a total of 550 chips test positive, we assign probability P(Positive) = 0. 55. Similarly, P(Inconclusive) = (150+75)/1000 = 0. 225, P(Negative) = (75+150)/1000 = 0. 225. Figure 20. Expressing Prior and Likelihoods as Frequencies 0. 550 positive … test 0. 225 inconclusive … 0. 225 negative … 242 Chapter 20 Value of Imperfect Information Of 550 chips that test positive, 525 are good chips. So if we observe a positive test result and decide to ship as is, the probability that the chip is good is P(Good|Positive) = 525/550 = 0. 9545. The other probabilities for the test alternative can be determined in a similar way. 20. 3 DECISION TREE MODEL The decision tree shows all probabilities based on the previous calculations. Figure 20. Decision Tree Model A B C D 1 EVSI 2. 25 2 $ 3 4 5 6 7 No add’l info 8 9 10 11 12 13 14 15 16 17 18 19 20 21 22 23 2 24 $27. 25 25 26 27 28 29 30 31 32 33 34 35 36 37 Test 38 39 40 41 42 43 44 45 46 47 48 49 50 51 52 53 54 55 56 57 58 59 E F G H I J K L 0. 7500 Good M N O P Q R S $40. 00 Ship as is $25. 00 0. 2500 Defective -$20. 00 1 $25. 00 EVUU Rework first $10. 00 $10. 00 0. 9545 Good $40. 00 Ship as is $37. 27 0. 5500 Positive 1 $37. 27 Rework first $10. 00 $10. 00 0. 6667 Good $40. 00 Ship as is $20. 00 0. 2250 Inconclusive 1 $27. 25 EVSP $20. 0 Rework first $10. 00 $10. 00 0. 3333 Good $40. 00 Ship as is $0. 00 0. 2250 Negative 2 $10. 00 Rework first $10. 00 $10. 00 0. 6667 Defective -$20. 00 -$20. 00 $40. 00 0. 3333 Defective -$20. 00 -$20. 00 $40. 00 0. 0455 Defective -$20. 00 -$20. 00 $40. 00 -$20. 00 $40. 00 The expected value using the imperfect test is $27. 25. This result is called EVSP = Expected Value using Sample Prediction or the expected value using imperfect information. EVSP is always calculated assuming the cost is zero, i. e. , assuming the information is free. 20. 4 Frequencies in Tables 243
To determine the value of the imperfect information, we see how much better it is compared to making the decision under uncertainty. This value is called EVSI = Expected Value of Sample Information or EVII = Expected Value of Imperfect Information. In general, EVSI = EVSP – EVUU, and here EVSI = $27. 25 – $25. 00 = $2. 25. We should be willing to pay a maximum of $2. 25 for this imperfect test. Figure 20. 9 EVPI and EVII Sensitivity to Prior Probability $16 $14 $12 Value of Information $10 $8 $6 $4 $2 $0 0. 0 0. 1 0. 2 0. 3 0. 4 0. 5 0. 6 0. 7 0. 8 0. 9 1. 0 Prior, P(Good) EVSI Base Case EVPI 20. FREQUENCIES IN TABLES The frequency calculations can also be arranged in table form. Figure 20. 10 Joint Outcome Table Test Result Positive Inconclusive Negative Component Condition Good Defective Consider the random process where we select a component at random. There are six possible outcomes, where an “outcome” is the most detailed description of the result of a random process. Here an outcome is described by the test result and component condition, represented by the six cells shown in the table. 244 Chapter 20 Value of Imperfect Information Figure 20. 11 Component Condition Frequencies
Test Result Positive Inconclusive Negative Component Condition Good Defective 750 250 For 1,000 chips, we expect 750 Good and 250 Defective. Figure 20. 12 Joint Frequency for Good Chips Test Result Positive Inconclusive Negative Component Condition Good Defective 525 150 75 750 250 For the 750 Good chips, based on the probabilities 0. 7, 0. 2, and 0. 1, we expect the 750 chips to have test result frequencies of 525, 150, and 75. Figure 20. 13 Joint Frequency for Good and Defective Chips Test Result Positive Inconclusive Negative Component Condition Good Defective 525 25 150 75 75 150 750 250
Similarly, for the 250 Defective chips, based on the probabilities 0. 1, 0. 3, and 0. 6, we expect the 250 chips to have test result frequencies of 25, 75, and 150. Figure 20. 14 Joint Frequency Table with Row and Column Totals Test Result Positive Inconclusive Negative Component Condition Good Defective 525 25 150 75 75 150 750 250 550 225 225 Figure 20. 15 Joint Probability Table with Row and Column Totals Test Result Positive Inconclusive Negative Component Condition Good Defective 0. 525 0. 025 0. 150 0. 075 0. 075 0. 150 0. 750 0. 250 0. 550 0. 225 0. 225 1. 000 20. Spreadsheet Revision of Probability 245 20. 5 SPREADSHEET REVISION OF PROBABILITY These screenshots show how the probability calculations can be arranged in a worksheet. The label “Main” refers to the main event of interest that affects the outcome values, i. e. , whether a chip is Good or Bad. The label “Info” refers to the result of imperfect information, i. e. , whether the test result is Positive, Inconclusive, or Negative. Figure 20. 16 Display 1 2 3 4 5 6 7 8 9 10 11 12 13 14 15 U Prior Likelihood Positive Inconclusive Negative Joint Positive Inconclusive Negative Posterior
Positive Inconclusive Negative V 0. 75 Good 0. 7 0. 2 0. 1 Good 0. 525 0. 150 0. 075 Good 0. 9545 0. 6667 0. 3333 W X Y 0. 25 = P(Main) Bad 0. 1 = P(Info | Main) 0. 3 0. 6 Bad Preposterior 0. 025 0. 550 = P(Info) 0. 075 0. 225 0. 150 0. 225 Bad 0. 0455 = P(Main | Info) 0. 3333 0. 6667 Figure 20. 17 Formulas 1 2 3 4 5 6 7 8 9 10 11 12 13 14 15 U Prior Likelihood Positive Inconclusive Negative Joint Positive Inconclusive Negative Posterior Positive Inconclusive Negative V 0. 75 Good 0. 7 0. 2 0. 1 Good =V$1*V3 =V$1*V4 =V$1*V5 Good =V8/$X8 =V9/$X9 =V10/$X10 0. 25 Bad 0. 1 0. 3 0. Bad =W$1*W3 =W$1*W4 =W$1*W5 Bad =W8/$X8 =W9/$X9 =W10/$X10 W X = P(Main) = P(Info | Main) Y Preposterior =SUM(V8:W8) =SUM(V9:W9) =SUM(V10:W10) = P(Info) = P(Main | Info) 20. 6 GENERAL PROBABILITY CONCEPTS An outcome is the most detailed description of the uncertain result of an outcome-generating process. An event is a collection of outcomes. An event may be a single outcome. 246 Chapter 20 Value of Imperfect Information The following general probability concepts are discussed using the event of a Good component and the event of a Positive test result from the Technometric example.
A simple probability is the probability of a single event. For example, P(Good) = 0. 75. A joint probability P(A) is the probability that event A and event B both occur. The occurrences of both event A and event B are uncertain. Joint probabilities do not usually appear on a decision tree. A joint probability is sometimes called the probability of the intersection or the probability of the simultaneous occurrence of two events. For example, P(Positive) = 0. 525. A conditional probability P(A|B) is the probability of event A conditional on event B, and by definition, P(A|B) = P(A)/P(B).
Outcome B, sometimes called the “conditioning” event, is known to have occurred or is assumed to have occurred. The occurrence of event A is uncertain. Sometimes we say simply that P(A|B) is the probability of A given B. For example, P(Positive|Good) = 0. 7. 20. 7 BAYES PROBABILITY CONCEPTS “Main” represents an event of the main uncertainty that affects payoffs. In the Technometrics problem, the two Main events are Good component and Defective component. “Info” represents an event of the information-gathering activity. In the Technometrics problem, the three Info events are Positive, Inconclusive, and Negative test result.
P(Main), called a Prior probability in Bayes terminology, is the simple probability of a Main event before any additional information is obtained. This probability is used to determine Expected Value of Perfect Information. For example, P(Good) = 0. 75. P(Info|Main), called a Likelihood, is the conditional probability of obtaining an Info event, assuming that a Main event has occurred. This probability is used to specify the relationship between an Info event and a Main event, thereby measuring the “accuracy” of the informationgathering activity.
Likelihoods do not usually appear on a decision tree. For example, P(Positive|Good) = 0. 7. Referring to the general definition of conditional probability, the joint probability can be calculated as P(A) = P(A|B)*P(B). For example, P(Positive) = P(Positive|Good)*P(Good) = 0. 7*0. 75 = 0. 525. P(Info), called a Preposterior, is the simple probability of an Info event. This probability is calculated by summing joint probabilities. The preposterior is sometimes called the probability of the union of two or more events.
For example, P(Positive) = P(Positive) + P(Positive) = 0. 525 + 0. 025 = 0. 55. P(Main|Info), called a Posterior, is the conditional probability of a Main event, assuming that an Info event has occurred. For example, P(Good|Positive) = P(Good)/P(Positive) = 0. 525/0. 55 = 0. 9545. 20. 7 Bayes Probability Concepts 247 Figure 20. 18 Formulas for Revising Probabilities P( Main ) Bayes “Prior” P( Info | Main ) “Likelihood” Inputs Multiply P( Info & Main ) “Joint” Sum Intermediate Calculation
Divide P( Info ) “Preposterior” P( Main | Info ) “Posterior” Outputs Bayes Rule is a formula that shows how the posterior probability can be computed from the priors and likelihoods, without explicitly identifying the intermediate computations of the joint probabilities and preposteriors. By the definition of conditional probability, an example of a posterior is P(Good|Positive) = P(Positive) / P(Positive) The simple probability P(Positive) can be expressed as the sum of the joint probabilities for the two ways that the event Positive can occur.
P(Good|Positive) = P(Positive) / [P(Positive)+P(Positive)] Applying the definition of conditional probability again, each joint probability can be expressed as the product of a conditional probability and a simple probability. P(Good|Positive) = P(Positive|Good)*P(Good) / [P(Positive|Good)*P(Good)+ P(Positive|Defective)*P(Defective)] This last expression, written to show how the posterior output is a function of the prior and likelihood inputs, is an example of Bayes Rule. 248 Chapter 20 Value of Imperfect Information 20. 8 BAYES REVISION BY FLIPPING PROBABILITY TREES 1.
Draw a probability tree with the main event branches on the left and the info event branches on the right. 2. Put the prior probabilities on the main event branches. 3. Put the likelihoods on the info branches. 4. Multiple each prior times each likelihood, and put the joint probability at the end of the tree on the far right. After completing the first four steps, the probability tree showing the input data and the calculated joint probabilities is shown below. Figure 20. 19 Probability Tree for Inputs and Joint Probabilities 0. 7 0. 525 positive 0. 75 good 0. 2 0. 150 inconclusive 0. 1 0. 075 negative . 1 0. 025 positive 0. 25 defective 0. 3 0. 075 inconclusive 0. 6 0. 150 negative 20. 8 Bayes Revision by Flipping Probability Trees 249 5. Draw a probability tree with the info event branches on the left and the main event branches on the right. 6. Put the joint probability at the end of the tree on the far right. Note that the order is different from the input tree, but each joint probability corresponds to a unique combination of an info event outcome and a main event outcome. 7. Sum the joint probabilities at the end of the tree to obtain the probability of each info event outcome on the far left.
For example, sum 0. 525 and 0. 025 on the far right to obtain 0. 550 on the far left. 8. To calculate the conditional probability of the main events (posterior probabilities), divide the joint probability on the far right by the probability on the far left (preposterior). For example, 0. 525 divided by 0. 550 equals 0. 9545. Figure 20. 20 Probability Tree for Outputs of Bayes Revision 0. 9545 0. 525 0. 550 positive 0. 0455 0. 025 defective good 0. 6667 0. 150 0. 225 inconclusive 0. 3333 0. 075 defective good 0. 3333 0. 075 0. 225 negative 0. 6667 0. 150 defective good